Nature:加州大学发布测定酿酒酵母中的单细胞蛋白丰度新方法
美国加州大学洛杉矶分校的一个研究组研发了一种新的eQTL标绘(mapping)方法,该新方法依靠GFP(绿色荧光蛋白)标记来测定酿酒酵母中的单细胞蛋白丰度。相关文章发表于2014年1月8日的《Nature》杂志上。
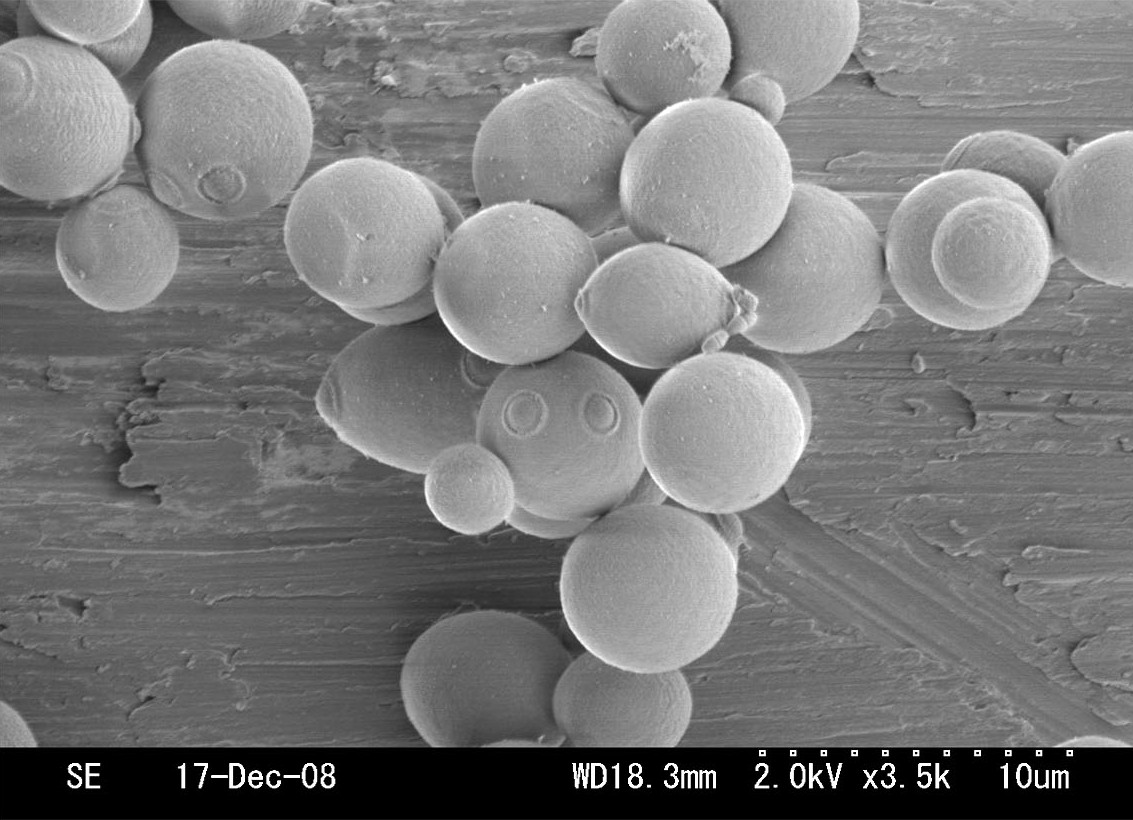
Nature:加州大学发布测定酿酒酵母中的单细胞蛋白丰度新方法
很多DNA变体通过改变一个或几个基因的表达水平来影响表现型,因此人们目前对标绘这些“表达量化性状位点”(eQTL)很感兴趣。
这篇论文介绍了一个新的eQTL标绘(mapping)方法,它设计用来克服现有方法的局限性(现有方法所关注的是RNA或蛋白丰度)。该新方法依靠GFP(绿色荧光蛋白)标记来测定酿酒酵母中的单细胞蛋白丰度。
然后,混合测序(Pooled sequencing)方法被用来对数千个蛋白丰度高和蛋白丰度低的人的整个基因组中的等位基因频率进行比较。
作者发现,对一个给定的基因来说,在影响mRNA和蛋白丰度的等位基因(位点)之间存在密切对应关系,同时他们也识别出了影响多个蛋白的热点位置——后者对基因调控网络有深远影响。
原文摘要:
Genetics of single-cell protein abundance variation in large yeast populations
Frank W. Albert, Sebastian Treusch, Arthur H. Shockley, Joshua S. Bloom & Leonid Kruglyak
Variation among individuals arises in part from differences in DNA sequences, but the genetic basis for variation in most traits, including common diseases, remains only partly understood. Many DNA variants influence phenotypes by altering the expression level of one or several genes. The effects of such variants can be detected as expression quantitative trait loci (eQTL). Traditional eQTL mapping requires large-scale genotype and gene expression data for each individual in the study sample, which limits sample sizes to hundreds of individuals in both humans and model organisms and reduces statistical power. Consequently, many eQTL are probably missed, especially those with smaller effects. Furthermore, most studies use messenger RNA rather than protein abundance as the measure of gene expression. Studies that have used mass-spectrometry proteomics reported unexpected differences between eQTL and protein QTL (pQTL) for the same genes, but these studies have been even more limited in scope. Here we introduce a powerful method for identifying genetic loci that influence protein expression in the yeast Saccharomyces cerevisiae. We measure single-cell protein abundance through the use of green fluorescent protein tags in very large populations of genetically variable cells, and use pooled sequencing to compare allele frequencies across the genome in thousands of individuals with high versus low protein abundance. We applied this method to 160 genes and detected many more loci per gene than previous studies. We also observed closer correspondence between loci that influence protein abundance and loci that influence mRNA abundance of a given gene. Most loci that we detected were clustered in ‘hotspots’ that influence multiple proteins, and some hotspots were found to influence more than half of the proteins that we examined. The variants that underlie these hotspots have profound effects on the gene regulatory network and provide insights into genetic variation in cell physiology between yeast strains.